UC Berkeley Undergrads Use Machine Learning — and Sharp Eyes — to Discover a New Asteroid
The Undergraduate Lab at Berkeley (ULAB) Physics and Astronomy Lab is a student-initiated, student-led program, sponsored by N3AS. ULAB researchers Supash Bhat, Gigi Flores, Alexandra Lu, Michelle Duan, Olivia Wagner, and Andrew Kesler (lead by student mentor Tanvi Batra) utilized machine learning to discover a new Near Earth Object, Asteroid “2025 FD.”
Modern astronomy is flooded with data, but buried in that deluge may lie threats to our planet. At UC Berkeley, a year-long research effort led by undergraduate students tackled a key question in protecting the earth: Is our current system for identifying near-Earth asteroids catching all viable candidates?
According to the undergraduate team, the answer is no. Their methodology for searching astronomical images led to the discovery of a new object: Asteroid 2025 FD.
The project was part of UC Berkeley’s Undergraduate Laboratory for Physics and Astronomy (ULAB), a student-led course supported by N3AS, that offers students an introduction to real-world research. ULAB students form groups of five or six, each mentored by a more senior undergraduate peer. Over the course of the year, each team conceives and proposes a project, learns the necessary background science and technical skills, carries out the research, and presents their results to the Berkeley community. The exposure to research fundamentals and ULAB’s collaborative environment helps students move on to work on research projects with Berkeley faculty.
This year, ULAB’s “Z-Streak” team also made notable contributions to astronomy. Analyzing images taken by Caltech’s Zwicky Transient Facility (ZTF) at Palomar Observatory, the team identified around 300,000 streaks of light in the sky each night. Most of these streaks are simply artifacts of the detection pipeline, so identifying genuine astronomical objects is like finding a needle in a haystack. To do so, the Z-streak team developed and applied machine learning and deep learning filters to reduce the pool to about 3,000, which they then scanned by eye, narrowing it down to 30 viable candidates.

Among these, they found that one streak could be confirmed to be real across multiple observations. Using orbital simulation tools, they calculated its trajectory and submitted their results to the Minor Planet Center, which approved the finding and officially designated the object Asteroid 2025 FD.
According to the Z-Streak’s team calculations, Asteroid 2025 FD will eventually pass as close as 818,000 miles from Earth. While it poses no immediate danger, its detection underscores the importance of tightening our screening methods. “We realized that even after the machine learning filters, a lot of the most promising streaks were still being missed,” said Supash Bhat, a member of the team. “So we proposed a new set of parameters and visual cues that weren’t accounted for in the current system—and that’s how we found 2025 FD.” In an era of vast astronomical datasets and an increasing number of transient events, the team’s work serves as a reminder: sometimes, it still takes a sharp eye to see what machines miss.
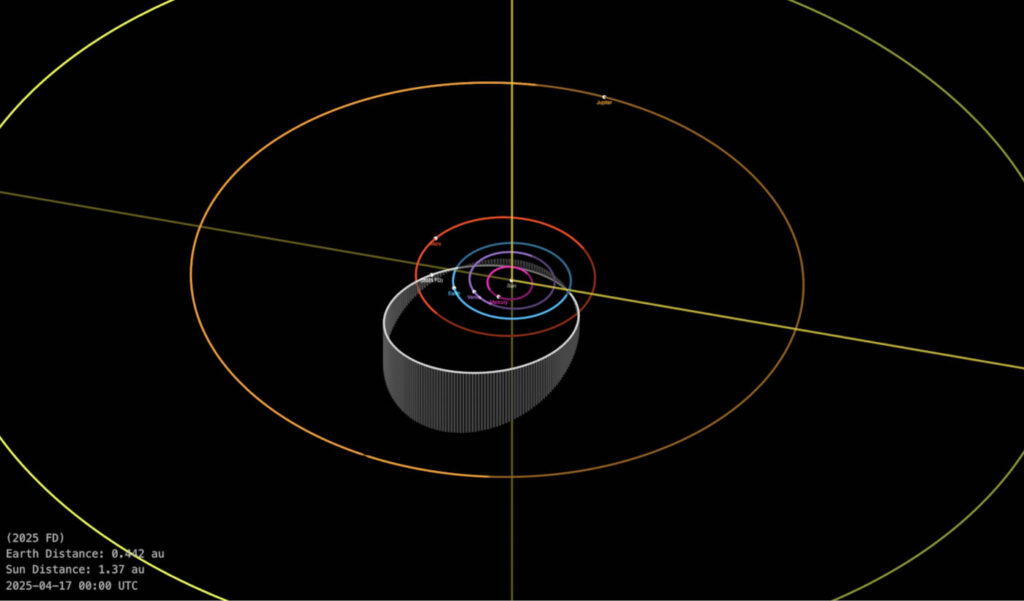
After confirming a consistent streak across multiple observations, we used orbital simulation tools, including Project Pluto’s Find_Orb, to determine the asteroid’s trajectory. The Minor Planet Center officially published our findings, and we received First Place at the ULAB Physics Research Fair for this work. The project highlights the effectiveness of combining AI tools with observational astronomy to accelerate NEO discovery and verification. — Supash Bhat